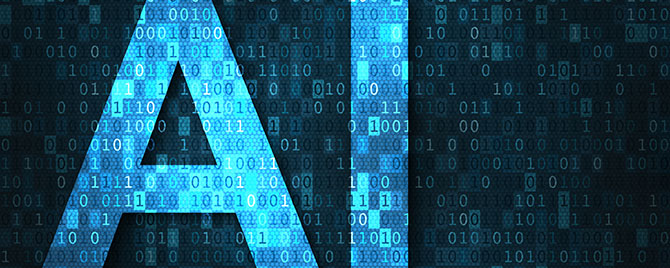
Last year, I shared about three emerging AI and Data Science trends for 2019. Firstly, we discussed how banks will begin to operationalize their data science pipeline by restructuring people, processes and technology. Secondly, we looked at how cross-industry data-sharing partnerships will be forged to support customer products and services. Finally, we also emphasized the need for regulations and information security policies to keep pace with the changing technology landscape.
There has been good progress in each of these areas over the past year, in turn shaping up a more conducive environment for the adoption of AI in the financial industry as we move forward into 2020.
Next Stages for the Data Science Pipeline and AGILE Workflows
Many financial institutions have spent the past year laying the architectural foundation for their data pipeline. Data lakes, typically built on a distributed storage system such as Hadoop Distributed File System (HDFS) or S3 Object Data Store, have been set up to consolidate a variety of data formats into a central repository.
The next stage of data pipeline implementation revolves around structuring a data warehouse on top of the data lake. The key challenge for most banks in setting up a data warehouse lies in ensuring data quality, which is time and resource intensive. Metadata needs to be labelled accurately and in a way that is relevant for the business’ needs. As data warehouse labelling is business case-centric, there are various strategies that banks can adopt to progressively migrate data into the warehouse. While banks may choose to ingest data for innovative projects into the warehouse first to minimize the impact to existing consumer-facing operations, it may be more meaningful to deploy projects with significant improvements to operational efficiency sooner than later to drive cost efficiencies and long-term business sustainability. Figuring out what data should move into the data warehouse first is a matter of the bank’s business strategy and risk assessment plan.
In the coming year, most banks would have established a foundation in data lake and data warehouse capabilities. This would naturally lead to more focused development of machine learning and AI techniques being deployed to support business needs across the banks.
Widespread adoption of data science techniques will also shift financial institutions increasingly towards AGILE workflows. Most banks have already fully adopted an AGILE approach for projects with limited connectivity to legacy systems. However, projects that require integration with legacy systems have continued to take on a semi-AGILE approach as data needs to be migrated from the legacy systems to the modern data stack. Moving ahead, there will be more thought given to onboarding legacy system projects onto a fully AGILE workflow where new systems are built with modular and scalable capabilities.
Cross-Industry Data Sharing Partnerships
It is heartening to observe the growth in government efforts to support cross-industry data sharing. The Data Collaborative Programme, led by Singapore’s IMDA and GovTech, provides a framework for managing data-sharing in a safe and sustainable manner. Coupled with this framework is a sandbox to encourage innovation and experimentation on proof-of-concepts for these cross-industry partners to test and learn before going to market.
With the issuing of digital bank licenses next year by Monetary Authority of Singapore’s (MAS), cross-industry data sharing is likely to gain more traction. New financial players will seek out cross-industry partnerships to widen their pool of data sources to support innovative ways of engaging with customers.
Governments have also begun to provide banks with access to a central repository of government-verified personal data services, which has helped to simplify product application submissions. For instance, it has become the norm in Singapore where consumers use their SingPass authentication to provide banks with access to their personal income tax and Central Provident Fund (CPF) information to support their online credit card and home loan applications. Elsewhere in the region, the National Digital ID (NDID), a blockchain database initiative led by the Thai government, will allow banks in Thailand to access consumer’s personal information for electronic know-your-customer (e-KYC) as well as to apply for banking products. It will only be a matter of time when other countries in the region follow suit.
From Open Dialogues on AI Ethics to Validation Frameworks
The dialogues on AI and data ethics have led to positive developments, such as the need for governing principles and industry collaborations to support the responsible use of AI in the financial sector. Several countries such as the US, Australia as well as countries in the European Union have developed guidelines for AI governance in the private and public sector. In Southeast Asia, the MAS in Singapore has taken the lead with the release of a set of principles to promote fairness, ethics, accountability and transparency (FEAT) for the use of AI.
While setting principles provide financial institutions a good direction on how AI should be used, the discussion for the year ahead is likely to be centered on the development of a set of more specific guidelines for organizations to evaluate their AI solutions against FEAT principles. Veritas, a recently announced consortium driven by MAS together with the financial industry is created with this intention. The objective is to develop a framework and a suite of open source tools that can provide a verifiable way for the banking and finance sector to incorporate FEAT principles as part of their AI products. Appropriate governance around AI usage is critical in ensuring that AI applications are properly managed and do not pose any significant risk to consumers as well as the financial system as a whole. While there may be concerns that regulatory guidelines my hinder the adoption of AI, it is my belief that proper governance will instead give the industry the confidence and assurance on the application of AI driven decisioning to support and transform financial services.
So 2020…
The adoption of AI and data science will quicken in pace in the coming year in the financial industry. Banks and financial institutions that have set up their data lakes and data warehouses will start to develop in-house machine learning and AI capabilities to support business lines and enhance operational efficiency. Government initiatives such as experimental sandboxes and the issuing of new digital bank licences will pave the way for more cross-industry partnerships in data-sharing. With widespread use of AI and data science applications across the financial industry, there will be more discussions and industry collaborations on the responsible use of AI, so as to cultivate trust and confidence in AI driven decisions for financial services.
This article is also published on Learn@IBF.
Facebook Comments