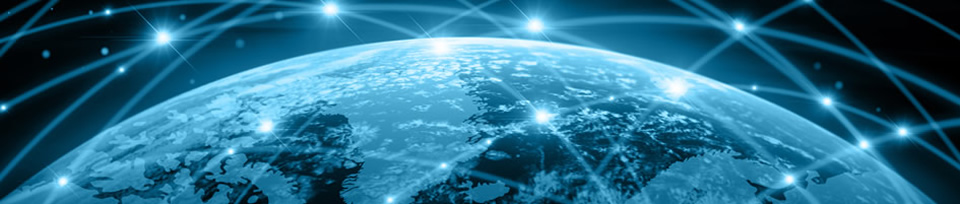
In the financial industry, the rapid growth of financial data has paved the way for data science and machine learning to become an integral part of solving business challenges. It has become pivotal in creating new profit centres and streamlining operational costs. As with all new practice fields, understanding and navigating the key challenges of data science will help businesses reap the returns from machine learning.
First, laying the foundation for a good data infrastructure is essential. New data capabilities must be well integrated with existing enterprise legacy systems. Most established firms face IT systems and processes that do not readily accommodate new sources of data and storage. In managing a progressive transition to a modern analytics end-to-end pipeline, it is important to design a “plug and play” infrastructure with modular components from ingestion, storage, processing analytics to visualisation. Modular components improve the sustainability of an enterprise system, so that it can be re-customised when future requirements change or when new technology is introduced. On top of this pipeline, APIs can be built to facilitate a more flexible platform for a community of data scientists and developers to create models and algorithms.
Next, adapting new data capabilities to business needs. How can enterprise systems make data readily accessible, easy to use and quick to deploy? Most banks still rely on mainframe systems, where data is collected in “batches” and periodically fed through the system in a process known as “batch processing”. While mainframes are equipped to deal with large amounts of data, it does not have the ability to perform real-time data analytics. Business users, who require real-time data analytics such as in fraud detection, will need a system with stream processing capabilities, where data is fed continuously into the system. Transitioning to a system with real-time data analytics is a challenge. A component for stream processing capabilities needs to be fitted in the pipeline, and open-sourced software such as Storm, Flume and Kafka may be explored.
Finally, the real value of data science lies in developing models for prediction. As businesses grow their data capabilities, they move beyond descriptive and diagnostic analytics to predictive analytics. Building training models that accurately predict for new data remains a balancing act. How do you avoid “overfitting” of training data? That is, a situation where the algorithm has modelled the training data too well, and may not produce the desired result when new data is fed. This is the turning point where data science shifts from a “science” to an art. Data scientists have to deftly calibrate their models to ensure they are not too complex. They will need to strike a balance in how they reduce the bias in their model at the expense of increasing variance. Well thought out models take a methodical approach in ensuring that each variable is given due and rigorous consideration.
Having a good grasp of these challenges for data scientists and business users will help firms move forward in building an effective data science strategy that can deliver on the promises of what machine learning can offer.
The content of this article was first presented at Machine Learning Asia Summit, March 2018 in Singapore.
Facebook Comments